The size of the AI market is more than $200 billion as of 2023 and is predicted to rise twentyfold by 2033. This can be explained by the increasing number of businesses adopting AI solutions, meaning that only technologically advanced companies will have a chance to stay in the near future. So, no wonder companies tend to find AI consulting partners to support them on their digital transformation journey. And in particular, this concerns non-technical businesses.
In this post, you’ll discover more about the benefits and types of AI consulting. You will also learn the entire AI implementation process and how to choose AI consultants for your project.
Benefits of AI Consulting
When engaging an AI consulting firm, C-level executives seek expertise to drive transformation. Here’s why partnering with a trusted provider is essential:
- Spotting opportunities: AI consultants identify viable AI use cases, enabling effective pilots and prioritizing impactful initiatives.
- Roadmap guidance: They oversee projects from ideation to deployment, estimate time and budgets, define team requirements, and structure phases for faster results.
- Data preparation: Consultants help curate, clean, and structure data, ensuring models are accurate and advising on data collection strategies. If you doubt how to proceed with gathering appropriate data, don’t hesitate to request assistance from professional AI engineers.
- Model testing: They validate models rigorously to ensure performance and reliability in real-world scenarios.
- Risk mitigation: With experience, they foresee challenges, offer solutions, and help avoid setbacks.
- Ethical assurance: Consultants ensure AI solutions are fair, transparent, and regulatory-compliant.
Even with in-house AI expertise, a tech partner boosts outcomes and minimizes risks, ensuring success in innovative projects.
Types of AI Solutions for Businesses
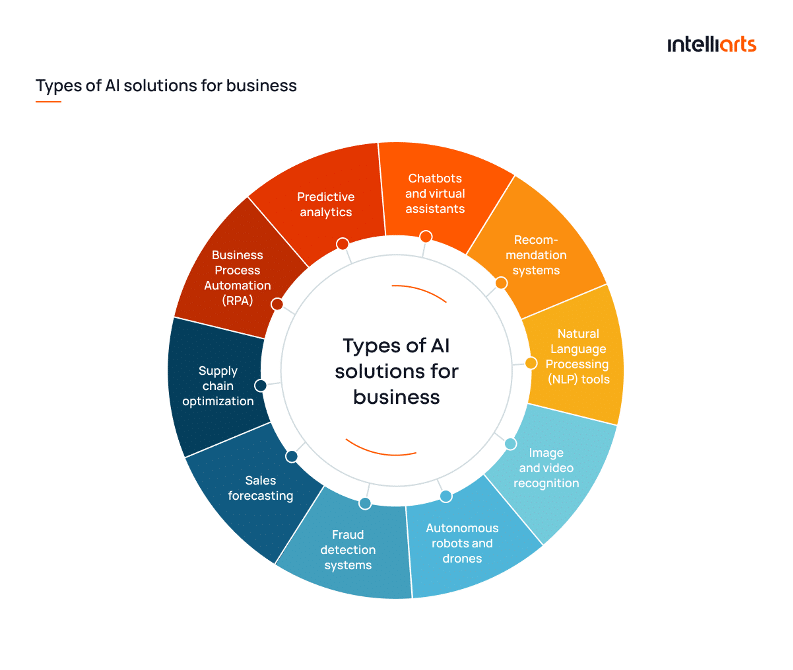
AI consultancy should serve a clear goal of advancing the business with advice on integrating one or another technology. Here are some of the AI solutions businesses may consider utilizing:
1. Predictive analytics
What’s this? Leveraging historical data to forecast future trends, businesses can anticipate customer needs or market shifts.
Examples: Forecasting stock demands, predicting market changes, customer behavior, or equipment failures.
2. Chatbots and virtual assistants
What’s this? Automated tools that handle customer queries, enhancing customer service efficiency.
Examples: Customer support chatbots on e-commerce sites that address common inquiries.
3. Recommendation systems
What’s this? Algorithms suggesting products or content based on user behavior.
Examples: Netflix’s movie suggestions or Amazon’s product recommendations.
You can learn how big data is used in Amazon’s recommendation system from the video below:
4. Natural Language Processing (NLP) tools
What’s this? Software that understands and generates human language, aiding in content analysis or creation.
Examples: Sentiment analysis tools gauging customer reviews or document data extraction.
5. Image and video recognition
What’s this? Technologies for identifying patterns within visual data.
Examples: Face recognition for security purposes or defect detection in manufacturing.
6. Autonomous robots and drones
What’s this? Machines capable of independent operation, optimizing task completion.
Examples: Drones for inventory management in large warehouses or robots assisting in surgeries.
7. Fraud detection systems
What’s this? Algorithms that can identify unusual patterns to detect and prevent fraudulent activities.
Examples: A banking system flagging suspicious transactions, telecom fraud detection, or Anti-Money Laundering (AML).
8. Sales forecasting
What’s this? Using AI to predict future sales, helping businesses to prepare and strategize.
Examples: E-commerce platforms anticipating holiday sales surges or manufacturing companies predicting seasonal demand.
9. Supply chain optimization
What’s this? AI-driven tools that ensure efficient product movement from manufacturers to consumers.
Examples: Predictive tools optimizing shipping routes or restocking schedules.
10. Business Process Automation (RPA)
What’s this? Software robots that perform repetitive tasks, increasing operational efficiency.
Examples: Bots entering data into CRM systems or automating invoice processing.
There are multiple other solutions, and more emerge over time. Having a consultation with a knowledgeable provider can shed some light on the actual prospects for your business, revealing the overlooked digitalization opportunities.
Selecting the Right AI Consulting Partner
When selecting a company that will serve as a driver of your digital transformation, it’s crucial to choose the right fit rather than just the most well-known company. Guide your choice with the following considerations:
- Expertise and experience. Evaluate the chosen consultant’s past AI projects to ensure they have the skill set tailored to your needs and are familiar with solutions similar to the one you’re keeping in mind.
- Reputation and references. Assess the firm’s track record by seeking feedback from past clients or evaluating case studies. You may also consider exploring the Clutch platform or similar resources to gather customer reviews.
- Domain-specific knowledge. Ensure the consultant understands the nuances of your industry, which can be instrumental in crafting AI solutions that truly resonate.
- Costs and pricing structure. Balance the value the provider can bring to your business with their pricing policy.
- Flexibility and scalability. If you expect changes in the scope of the project as it goes, it would be better if you pick a consultant who can adapt to changing project requirements.
Most likely, you’ll end up having several suitable providers on your shortlist. The reasonable action here is to have an initial consultation with representatives of each company. Ask them the questions listed below to validate their expertise and fit.
- What previous AI projects have you worked on?
- Can you provide case studies or references?
- How do you handle data security and privacy?
- What is your pricing model?
- What is your perspective on the ethical use of AI?
Include other questions to address all uncertainties about companies you may have, gather additional information, or just make any significant matters clear. Choose AI consultants that are better suited to the intended project and your culture, maintain clear communication, have relevant expertise, and are generally the most trustworthy, and you’ll succeed.
Process for Implementing AI Consulting
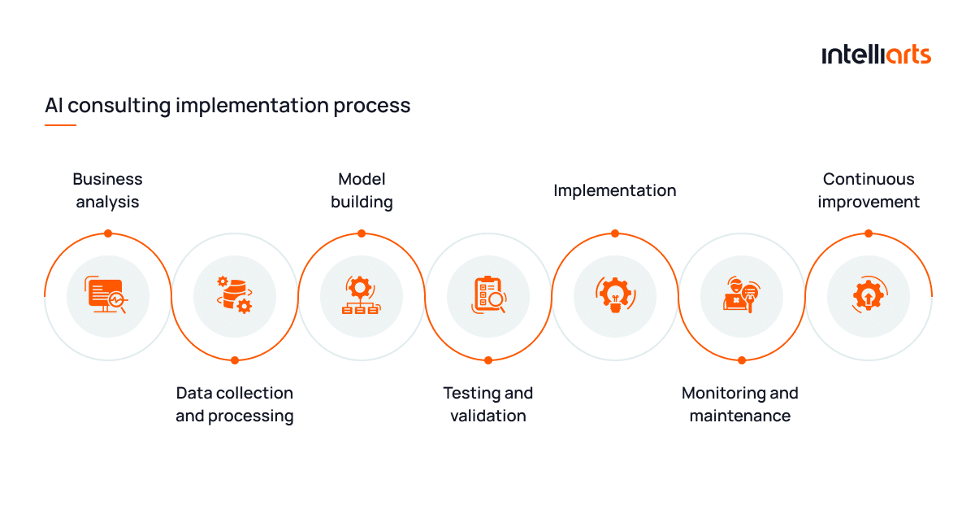
Now, let’s get to know the process for crafting an innovative solution or adopting an ML model into your workflow when partnering with an AI consulting company. The involvement of the invited vendor may vary from solely consulting impact to execution of some or even all implementation stages, which include the following:
#1 Business Analysis
The foundation of any AI-driven project starts with a comprehensive business analysis. This involves understanding the company’s objectives, current workflows, pain points, and potential AI opportunities. By collaborating closely with stakeholders, AI consultants can:
- Identify key areas where AI can have the most significant impact
- Provide a clear vision for the subsequent stages of the process
This phase is crucial for aligning the AI solution with the business goals and ensuring a meaningful transformation.
#2 Data Collection and Processing
Data is the cornerstone of creating and implementing AI solutions. In this stage, data from various sources is gathered, be it from internal databases, external platforms, or even IoT devices. Once collected, it undergoes a rigorous cleaning and preprocessing routine. Missing values are handled, outliers are identified, and data is transformed into a format suitable for model building. Proper data collection and processing ensure the AI models will be both reliable and accurate.
#3 Model Building
With clean data in hand, the AI consultancy delves into the construction of a machine learning model tailored to the business’s specific needs. This involves:
- Data utilization. Using cleaned data for model creation
- Algorithm selection. Picking the right algorithms for specific tasks
- Training phase. Teaching the model how to use the data
- Optimization. Adjusting for better performance
- Refinement. Continuous model tweaks for accuracy
Throughout this process, the model needs to be refined multiple times to ensure it captures the patterns and insights embedded in the data.
#4 Testing and Validation
Before deploying the AI solution in a live environment, it undergoes thorough testing. This step involves:
- Testing on a separate dataset
- Accuracy check
- A/B testing
- KPIs and business objectives evaluation
- Performance evaluation
- Issue identification
- Deployment preparedness
If the results are not satisfactory, there will be the need to repeat the steps of data collection and processing, model training, and model testing. When any discrepancies or issues identified during this phase are addressed, this ensures the model is ready for real-world challenges.
#5 Implementation
Once tested and refined, the AI model is integrated into the existing systems and workflows of the business. This phase may involve software development, infrastructure adjustments, and user training.
The main goal here is to ensure seamless operation, where the AI solution enhances the business processes without causing disruptions. It may take time to integrate the AI solution into the digital infrastructure, so for a business, it’s better to strategize for downtimes in advance.
#6 Monitoring and Maintenance
Post-implementation, it’s vital to keep a close watch on the AI solution to ensure it continues to operate as expected. Monitoring tools are put in place to track performance, and any anomalies or deviations are addressed promptly.
Maintenance might also involve periodic retraining of the model, especially if new data becomes available or if the business environment changes.
#7 Continuous Improvement
The world of AI is dynamic, with new advancements and techniques emerging regularly. As such, it’s essential for businesses to:
- Stay updated. Keeping abreast with AI advancements to timely detect the need for a new feature or any other modification
- Conduct model refinements as necessary. Undergoing stages of data preparation, training, and testing, based on new data or feedback
- Consider and implement feedback. Using real-world results to iterate
- Seek opportunities. Finding new areas for AI enhancement
By carrying out these activities businesses can ensure their newly introduced AI solutions will have an extended lifecycle and stay relevant for a longer period.
Are you looking for expert development assistance? Contact Intelliarts and let our team of AI engineers contribute to your best project.
Final Take
In this post, we had AI consulting explained in great detail. This service line comes in use whether you own a technology company yourself or don’t have in-house expertise in AI and ML. Your AI partner will guide you through the entire IT solution implementation process and ensure your investments in your project will bring you an increase in revenue.
FAQ
- Are there any common challenges or obstacles when implementing AI consulting?
These include data quality issues, resistance to change, unrealistic expectations, lack of domain-specific expertise, and integration complexities with existing systems.
- Can I start with AI consulting if my business is just starting out and has a limited budget?
Absolutely. Many AI consulting agencies offer scalable solutions tailored to startups or businesses with budget constraints. Besides, requesting AI consulting proves cost-effective compared to DIY development of an AI-based solution.
- How do I measure the Return on Investment (ROI) of AI consulting services for my business?
Calculate the tangible improvements in efficiency, revenue growth, cost savings, and customer satisfaction against the investment made in consulting services to measure the return for every dollar invested.