Machine learning (ML) model monitoring refers to the ongoing process of tracking, analyzing, and assessing the performance of ML models to ensure they continue to meet expected outcomes. This practice is critical for maintaining accuracy, reliability, and consistency, especially in cloud-based environments where resources are dynamic and models may evolve over time.
Effective monitoring is crucial for B2B businesses in the machine learning sector. It allows them to detect issues such as data drift, concept drift, or degraded performance, which could otherwise lead to flawed predictions or business disruptions. In a cloud context, monitoring helps ensure that ML models operate smoothly and efficiently, supporting the scaling and flexibility required by businesses with varying workloads. By monitoring ML models in the cloud, B2B companies can maintain a competitive edge and ensure the integrity of their machine learning projects.
Challenges in Monitoring ML Models in the Cloud
Monitoring ML models in a cloud environment presents unique challenges that require careful attention. One of the most significant issues is data drift, which occurs when the input data used to train the model changes over time, leading to reduced accuracy. This can happen due to evolving trends, new data sources, or seasonal variations.
Concept drift is another challenge, where the underlying relationships between features and outcomes change. This shift can render a model’s predictions less reliable, requiring retraining or model adjustment. Monitoring is essential to detect these changes early and take corrective action.
Maintaining performance under variable workloads is also a key challenge. As businesses scale their operations, ML models may need to handle larger datasets and more complex computations. This can strain resources and lead to slow response times or decreased accuracy. The Nebius GPU cloud provider addresses this challenge by offering scalable resources and high-performance computing, ensuring that ML models remain efficient and responsive.
To overcome these challenges, businesses must implement robust monitoring strategies that include real-time analytics, anomaly detection, and regular model validation. By doing so, they can ensure the continued success and reliability of their ML projects in the cloud.
Key Strategies for Effective ML Model Monitoring
Successful ML model monitoring in the cloud requires a combination of real-time tracking, robust analytics, and continuous validation. Real-time analytics is crucial for monitoring ML models, allowing businesses to track performance metrics as they evolve. This approach helps detect issues like data drift or concept drift early, enabling swift corrective measures.
Anomaly detection is another key strategy. By using advanced algorithms, businesses can identify unexpected behavior or outliers that might indicate a problem with the model’s predictions. This strategy enhances reliability by providing a mechanism for early warning and intervention.
Model validation plays an essential role in ensuring that ML models remain accurate and reliable. Regular validation tests the model’s predictions against known outcomes, providing a benchmark for performance. By implementing these strategies, B2B companies can ensure their ML models in the cloud remain effective, accurate, and adaptable to changing conditions.
Ensuring Scalability and Flexibility in Cloud Monitoring
Scalability is a cornerstone of effective ML model monitoring in the cloud. As workloads fluctuate, businesses need the ability to scale their monitoring resources accordingly. This scalability ensures that ML models can handle varying data volumes without compromising performance or accuracy. In the cloud, scalability is often achieved through auto-scaling features that adjust resources based on real-time demand.
Flexibility in resource allocation and cloud configurations further enhances monitoring effectiveness. Businesses can customize their cloud environments to suit specific monitoring requirements, allowing them to adapt to evolving workloads. Flexibility also extends to the choice of monitoring tools and technologies, enabling businesses to select the best solutions for their unique needs.
By ensuring scalability and flexibility, companies can maintain optimal performance in ML model monitoring. This approach allows them to respond to changing business requirements while keeping costs under control and minimizing downtime due to resource constraints.
Cost-Effective Approaches to ML Model Monitoring in the Cloud
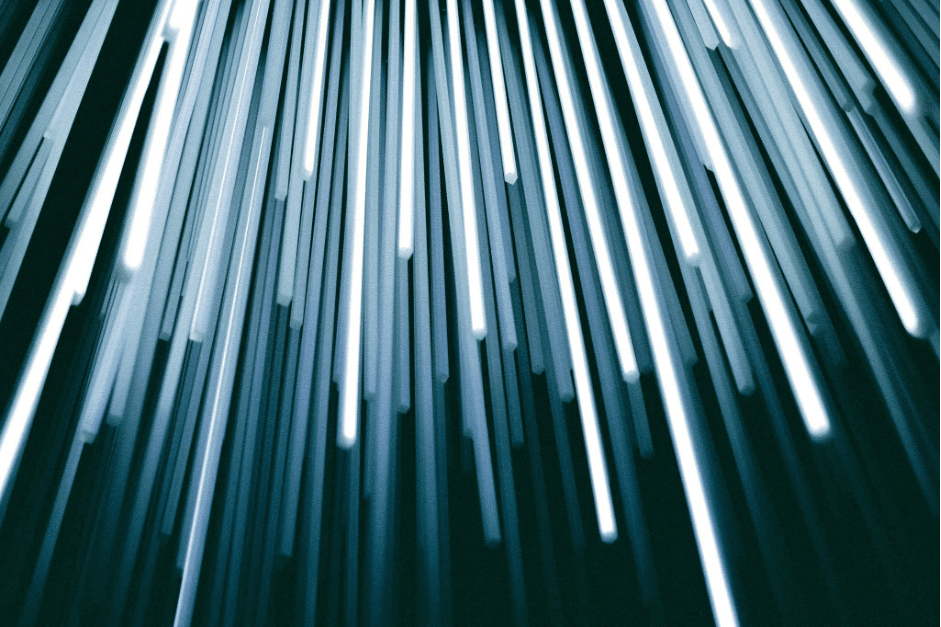
Cost efficiency is a critical consideration for B2B businesses monitoring ML models in the cloud. Different pricing models offer varying degrees of flexibility, allowing companies to choose the most cost-effective approach based on their needs. Pay-as-you-go pricing, for example, is ideal for businesses with fluctuating workloads, as it enables them to pay only for the resources they use.
Subscription-based models provide predictability, offering a fixed rate for a specified period. This stability helps businesses budget their cloud monitoring expenses more effectively. Reserved instances, which allow businesses to reserve computing resources at a discounted rate, are another cost-effective option, especially for companies with consistent monitoring demands.
Resource optimization techniques also contribute to cost efficiency. By employing load balancing, businesses can distribute monitoring tasks evenly across cloud resources, reducing bottlenecks and improving efficiency. Additionally, auto-scaling ensures that resources are allocated based on demand, preventing over-provisioning and reducing unnecessary costs.
These cost-effective approaches help B2B businesses strike the right balance between effective ML model monitoring and budget constraints in the cloud environment.
Security and Compliance Considerations for Cloud Monitoring
Security is crucial for monitoring ML models in the cloud, requiring measures such as encryption to protect data in transit and at rest. Access controls are essential, ensuring only authorized users can interact with sensitive information. Compliance with industry regulations like GDPR and HIPAA ensures that cloud monitoring aligns with legal requirements, safeguarding data privacy.
Tools and Technologies for ML Model Monitoring
Cloud-based ML model monitoring relies on a variety of tools and technologies. Dashboards offer visual insights into model performance, enabling teams to track key metrics in real time. Alert systems send notifications when anomalies are detected, allowing for prompt response. Cloud-based monitoring platforms provide a comprehensive suite of features for effective model tracking and analysis.
Conclusion
Effective ML model monitoring in the cloud involves strategies like real-time analytics, anomaly detection, and model validation. Scalability and flexibility are key for adapting to varying workloads, while security and compliance ensure data protection. As cloud-based monitoring evolves, it will continue to drive innovation in machine learning, offering B2B businesses effective solutions for maintaining and improving their ML models.